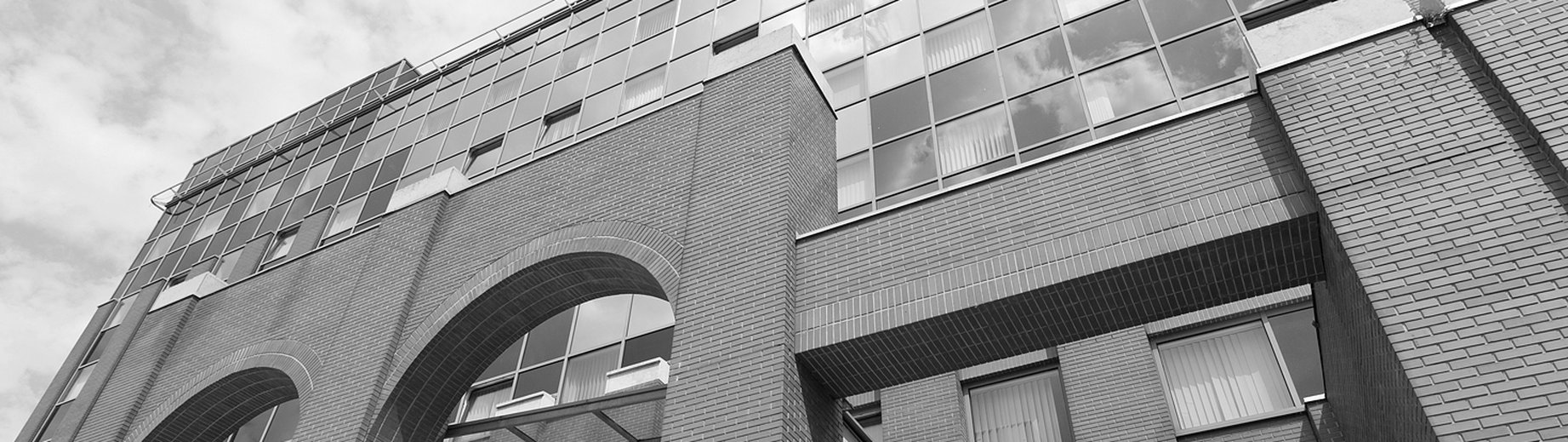
Abstracts
Abstracts
1. On the concept of quality for big data
Opening remarks
Tamás Rudas
Faculty of Social Sciences, Eötvös Loránd University, Budapest
Official statistics and survey methodology both work with well defined concepts of quality of data and a number of related best practices. These concepts are summarized and it is shown that they cannot be directly applied to big or organic data. Futher, the amalgamation paradox is disucssed, which seems to present an obstacle for applying internal consistency and related resampling approaches to defining quality for big data.
2. A possible approach to assess the validity of big data
Invited contribution
Anuška Ferligoj
University of Ljubljana and NRU HSE, Moscow
According to the ISO 9000 (2015) definition of quality, data quality can be defined as the degree to which a set of characteristics of data fulfills requirements which are: completeness, validity, accuracy, consistency, availability and timeliness. In the survey methodology the reliability and the validity is considered as the dimensions of the quality of data. The reliability refers to the extent to which the variance of the observed variable is due to systematic sources rather than “noise”. Therefore, it is defined as the ratio of variance in a variable due to nonrandom sources to the total variance of the variable (Lord and Novick, 1968). To assess the reliability measures of stability and measures of equivalence are used. The general definition of the validity is that it indicates the degree to which an instrument measures the construct under investigation (Bohrenstedt, 2010). There are several types of validity, e.g. criterion-related, content and construct validity. For the big data the criterion-related validity could be used. Here the correlation between the measured variable and a criterion variable, for which we assume that the measured variable should be related, is estimated. A type of this validity assessment uses the known group technique. If we know that certain groups vary on a variable of interest, differences between them can be used to validate it.
In the case of big data, the known group technique to assess the validity of a measured variable can be used if the data can be aggregated to certain aggregated categories (e.g. territorial units as countries or smaller units) for which there are known differences of the aggregated values between groups
3. Improving inference using low-quality data: the role of double sampling
Invited contribution
Ori Davidov
University of Haifa
In many situations, it is either infeasible or too expensive to collect high-quality data on the true outcome of interest from all sampling units. However, if an easy to collect proxy for the true outcome exists then a double sampling design may be beneficial. In such situations, there typically is a large primary sample on which the proxy data is available for all sampling units. On a random subsample of units from the primary sample, the true outcome is ascertained. This subsample is commonly referred to as the validations sample. The full data is then used to estimate the parameter of interest. In this talk we propose two approaches to empirically estimating a distribution function, and consequently its functionals, under double sampling. The theoretical properties of the proposed estimators are investigated and simulation experiments presented. Extensions to censored data and multivariate distributions are discussed.
4. Analyzing the Joint Dynamics of Survey-Event Network Data
Invited contribution
Christoph Stadtfeld
Swiss Federal Institute of Technology in Zurich
Dynamic social network data typically take one of two forms. The first type are traditional network panel data that consist of sequences of static network snapshots. They are useful to investigate change in self-reported and often not directly observable social networks, such as friendship perception networks. The second type are relational event data that represent time-stamped traces of dyadic data to investigate change in social networks of interactions, transactions, or spatial proximity. The first type is often collected through traditional survey techniques (survey data), and best practices have evolved over the years to ensure data quality. The second type can be collected automatically, for example, through online platforms or social sensors (event data).
The increasing availability of event data promise new opportunities to study social processes at a finer granularity and on larger scales. However, a number of sociological theories are not merely concerned with interactions, transactions, or proximity, but simultaneously with individuals’ perceptions of the social networks that they are embedded in. It is thus unclear whether meaningful insights can be derived from the study of event data in isolation.
The talk compares the two approaches in terms of sociological theories, data sources, and available methods of statistical analysis, and argues that data quality and empirical research designs can be improved by simultaneously considering them in a unified framework.
5. Same or different? Comparing Facebook data with survey answers on music taste and leisure time habits
Zoltán Kmetty and Renáta Németh
Hungarian Academy of Sciences, CSS-Recens Research group and Faculty of Social Sciences, Eötvös Loránd University, Budapest
Internal validity is one of the key criteria for assessing empirical researches. Both survey data and social media data have their limitations from this aspect. Surveys create an artificial questioning situation resulting in validity weaknesses. Among the issues, we can mention the self-reported nature of the data or the fact that the beliefs of the researcher can be reflected in the selection and wording of the questions (Groves et al, 2009). Validity of social media data is also challenged in many ways. Shifts in algorithms or user behavior introduce artefact patterns in data, while analysis tools like sentiment analysis methods used to measure emotions may constitute further source of bias (Panger, 2016). However, we can assume, that people’s “true” behavior can be monitored through direct observation more validly than in a self-reported way.
Systematic comparison of social media data and survey data is rather difficult, as there are no joint data available from both sources. In our paper we introduce a novel research where Facebook and survey data were collected from the same respondents. 150 respondents participated in this research, from East-Hungary, in 2019. We asked them to download their own Facebook data and share it with us, and also filled a 20 minutes self-administrated online questionnaire. In this questionnaire we asked series of questions about their leisure time activities, music taste, political preferences and other topics.
Our paper is a first step in our research comparing Facebook and survey data. We examine how political news consumption bubbles presents in Facebook data, and compare it with the survey answers of the same respondents. We focus on those attributes of the respondents which explain the differences of Facebook and survey-based data. We also map those methodological aspects of Facebook data which has to be considered before any measurement calculation.
We hope our research has a general methodological importance to reveal validity issues and to better understand the reasons behind the differences of survey and social media results.
Acknowledgement: Our research was supported by National Research, Development and Innovation Fund under grant NKFIH-FK128981.
6. Spatiotemporal Dimensions of Urban Mobility
Rafiazka Millanida Hilman
Central European University, Department of Network and Data Science
Mobility at the individual level is quite diverse, due to psychological, behavioral, and external conditions affecting each person. Individual mobility is dictated by one’s meaningful places like home and work locations, or frequently visited places for shopping, children activities, leisure, etc. The choice of these places is strongly determined by an individual’s socioeconomic status, which together with homophily stratifies society and leads to urban segregation.
In the past, travel survey conducted by Central Bureau of Statistics or Department of Transport served as the sole instrument in collecting information about personal travel within country. This survey records journeys on a given day in which origin and destination points with timestamps along with travel purpose included. In the case of London, London Travel Demand Survey is conveyed annually to 8,000 randomly selected households. There are questionnaires carried out comprising basic demographic information of household, further demographic and travel-related information of household member, and all trips made by every household member.
In the recent year, the disruption of digital technologies in transportation sector drives the emergence of New and Emerging Data Forms (NEDF) such as smart card. In London, Oyster card was issued for the first time in 2003. In 2012, the coverage of card use was already up to 80% of all journeys on public transportation network. Although in the beginning mainly it aims at optimizing fare collection systems, the information gathered starts to expand, covering card/subscription type, travel date, and origin-destination time stamp.
It is the interest of this research to bring rigorous assessment between the two mobility statistics. The first part of this research discusses the quality of data based on completeness, uniqueness, timeliness, validity, accuracy, and consistency. It responds to the following scopes: i) to what extent the current big data record differs from conventional method of organic data collection, ii) whether the gap between the two affects the data quality, iii) what kind of methods should be proposed to check and improve data quality.
The second part provides empirical ground by investigating the mobility of urban commuter in London. Data with 2,623,487 records are extracted from smart transportation card namely Oyster Card during November 2009. Moreover, the housing price is exerted to infer socioeconomic status of commuters. In order to reveal embedded pattern, predictive analysis is constructed by applying machine learning technique which is classification model. Accuracy score is recorded to measure prediction given by test data and evaluation generated by test label. Furthermore, predictive performance of the model could be improved by enforcing robustness test.
There are two approaches to take into account. The first is to identify the machine learning algorithm that is best-suited for the problem such as hold-out validation and cross validation. The second is to select the best performing model from a given hypothesis space by comparing the model performance on different attributes, for example time window (weekend and weekdays), housing value, and mobility pattern. It is expected that the quality of data could be enhanced, so does the validity of analysis, by running necessary procedures since data-preprocessing stage.
7. The Same but Different: Missing Ties of the International Trade Network
Alina Vladimirova
Institute of Oriental Studies of the Russian Academy of Sciences
Whether we deal with conventionally designed data from traditional sources or with new types such as organic data, the critical need for its’ quality control doesn’t change. It is even going stronger with a break of the replication crisis in science and the spread of the modern understanding of data value for actors on all levels of global systems. Now international organizations that are facing more complex challenges such as effective achievement of the Sustainable Development Goals (the SDGs) are conducting major revisions of data management practices and available data sources. Among them, trade statistics are considered as one of the most important and the most advanced in terms of data quality. On the one hand, this assessment is correct, as international organizations and national statistical services have been improving the methodology of these data collection and analysis for decades and now we have datasets such as “UN Comtrade” which contains well over 3 billion records since 1962 for over 170 reporter countries and areas. On the other hand, there are still numerous data quality problems that can lead to a reduction in the precision of estimated parameters and to an increase of the potential for bias. Considering that we were interested in accessing these data quality for international trade network models, we focused on issues connected to missing data and to the asymmetries of mirror statistics. Using the ISO standardized and the FAIR Data Principles approaches we have conducted a four stages research project starting with data quality diagnostics to practical recommendations on how to select a particular source for network analysis of the international trade taking into account missing ties and discrepancies in the statistics.
8. Searching for the truth by missing data – using administrative data for migration research
Vanda Kádár and Dávid Simon
Faculty of Social Sciences, Eötvös Loránd University, Budapest
In our presentation we outline our research trials on Hungarian migrant population abroad focusing mainly on the usage of Hungarian administrative database (Panel of Administrative Data - Databank of the Research Centre for Economic and Regional Studies, Hungarian Academy of Sciences, current status unknown). While a previous study about migration of Hungarian medical doctors based on the same database gave an acceptable approximation of the population, in case of the larger target population we faced more problems. In our presentation we sum up the measurement strategy of Hungarian migrants abroad and the problems we faced with. As a conclusion we summarize some possible limitations of the usage of administrative databases.
9. Spotlight on electronic repair – a transdisciplinary data quality evaluation towards its impacts on current challenges and future potentials for the repair sector
Eduard Wagner and Melanie Jaeger-Erben
TU Berlin
In recent years the vast promotion of big data and artificial intelligence told a narrative of immediate new possibilities for the circular economy that just need to be mined. The attention on those creative benefits often overlook their fundament, the data quality. It is argued that due to a lack of defined data strategies, the vast sprout of “data seeds” at various locations, qualities and formats lead to an organic growth of opaque “big data jungles”. This applies for manually (e.g., user generated) as well as autonomously (e.g., sensors) collected data. Traditionally, barriers to a more profound research on and with this data arise from company worries about liability, privacy and security issues, as well as uncertain benefits of distributed responsibilities for the single data sets.
One task of the “Chair for Transdisciplinary Sustainability Research in Electronics” at the TU Berlin, Faculty of Electrical Engineering and Computer Sciences, is to explore data jungles from a socialecological perspective and seek ways to use data mining expertise for sustainable business transformations. Especially the evaluation and utilization of historic data sets from industry is focused. The goal is to create synergetic added value from the provided data by simultaneously pursuing both, the individual case study bound problem from industry and the socio-ecologic research question. The research challenge lies in interdisciplinary research-industry corporation with individual goals and different resources, domain specific for electronic products.
Common data analysis approaches like KDD, Data mining and in particular Crisp-DM mostly focus on business intelligence alone. Meanwhile, social-ecological research could benefit from a more intense utilization of data mining methodologies and strategies. A systemic three step approach is proposed that combines different approaches to discover, develop and deliver knowledge from raw industrial data. Within the discovery stage, initially data quality is evaluated using a developed method based on
problem oriented data mining approaches and data quality evaluation guidelines. These include assessment guidelines, such as FAIR principles postulated by the GoFAIR initiative (used in Human Brain project) which provides data quality characteristics for multi-source historic “usable research data” including findable, accessible, interoperable and re-usable data. Dimensions as completeness, objectivity, relevancy, added value are considered and its relevancy is discussed.
This study focus on the quality evaluation step embedded in the “deliver” stage as the first part of the overall process. It was applied in a case study where repair data of household appliances were analyzed, in particular washing machines (28000 items), collected between 2003 and 2016 by an independent repair shop. Based on the available data and its quality, the following environmental impact related research question have been derived: can the lifetime be extended by applying ecodesign to avoid failure patterns and or reduce repair costs? Can a link between symptom and diagnosed broken part be established and systemized? The business oriented challenge was: can the evolution of total cost of ownership and profitability of repair be modelled and calculated? How can the data collection process and quality be improved?
Neglecting personal attributes/properties, the 2016 database consists of 20 attributes e.g., ID, brand, type, symptom, failure cause, repaired issue, repair time. While these attributes grew over time, an open text field was used more intensely at the beginning to describe further aspects like failure causes and repair actions, leading to unstructured free text and sparse data fields. Data cleansing was performed by a manually written rule based on python script to separate multi values in the comment field. Random sampling of the cleaned data shows that by an accuracy of 92% values where separated correctly and allocated to the right attributes.
To address data quality by means of consistency, a structured data format is proposed that can be used by repair industries, the open repair data alliance and repair lobby groups like the German NGO “Runder Tisch Reparatur” (round table repair) for dissemination to harmonize repair data collection. In conclusion, efficient data cleaning for historic data within the repair industry is essential to realize a harmonized data collection process and gain relevant insight.
10. The quality of big administrative data in biomedical research
Invited contribution
Tamas Ferenci
Physiological Controls Research Center, Óbuda University
So-called administrative (or financial) databases are increasingly used in the biomedical research. These databases, primarily collected to serve reimbursement purposes, present unique perspectives and challenges: on the one hand they offer unprecedented coverage both in time span and in the number of subjects covered, providing one of the few data sources that are truly "big" in clinical biostatistics, but on the other hand, they have inherent problems that stem from their primary purpose. Such data are prone to unintentional mistakes and clerical errors, but also to intentional falsifications, such as overcoding to increase funding. In my presentation, I'll briefly introduce this relatively novel data source and investigate the issues of this latter problem: how can we measure the quality of such data? (How can we define quality in this case at all?) How can we increase the reliability of studies based on such data sources?
11. The need for social turn in data science
Invited contribution
Zoltán Varju
Complytron
Data science has often been thought as a kind of science and as a kind of engineering. Practitioners tend to describe their activities as a rigorous scientific investigation via software engineering. As such, they are employing standard evaluation techniques on their models and software engineering methodologies on their code. Software testing, especially test-driven development, likens itself to Popperian falsification, while evaluation methodologies are thought to be analogous to the so-called scientific method. We will argue against these views since they are the main sources of unsuccessful data science projects because of the followings 1) This view takes data granted and it doesn't deal with the fact that gathering data is pre-determined by various factors 2) Software development is a social activity, as such, software expresses the shared knowledge of its creators and it assumes its users shares the same tacit knowledge as its creators. 3) Every software developer using a computer, a development environment, standard or third-party libraries has to make a leap of faith and accept their tools as correct ones. We have to mitigate these risks by employing best practices (e.g. MATTER for data gathering and annotation, CRISP-DM for process management) and by using open source solutions. Although the Popperian concept of science seems to be adequate at first glance, we have to learn the lessons from the philosophy of science and we have to face the external factors of our endeavor, we need a social turn.
Photo by Oleg Laptev on Unsplash.